
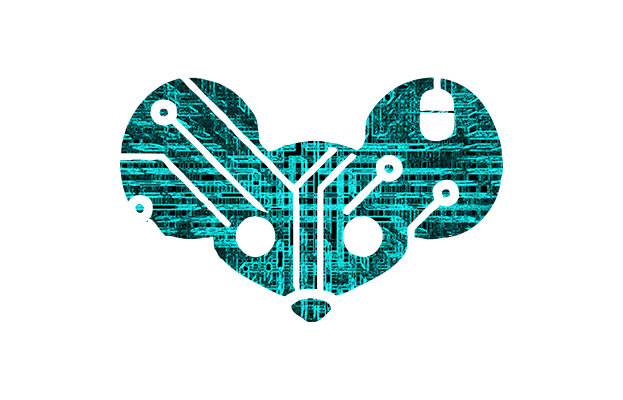
I used to do something like this before Signal became a thing. We used to use OTR via the Pidgin OTR plugin to send encrypted messages over Google Hangouts. Funnily enough, I’m pretty sure Pidgin supports Discord, so you could use the exact same setup to achieve what you described.
It was pretty funny to check the official Hangouts web client and see nonsensical text being sent.
No, the server is on the github account linked above as well. The repo is here.
Signal however doesn’t federate and does not generally support third-party clients.